In the frame of this WP, we set the basis towards the development of the different engineering and materials systems that will be developed in the project and will be integrated for testing and evaluation of the DOMMINIO concept. This WP has been completed and featured
- a description of ATL and FFF project manufacturing technologies,
- the preliminary design of novel equipment, such as a sensorized FFF 3D printed nozzle, a laser scanning heating system, and air/dry ATL NDT systems,
- a description of the magnetic disassembly process, as a new MRO methodology,
- the definition of testing procedures and quality monitoring systems, and
- project material selection, namely thermoplastic matrices for filament fabrication, cCNT fibres, magnetic nanoparticles, cCF and UD-tapes.
This WP outlines the end user project multi-functional case studies, an airframe access panel and a flight control spoiler structure. Additionally, a multi-objective-optimization framework was defined (Figure 1), aimed at optimizing the use-cases for mass, manufacturability, manufacturing quality, SHM, life cycle and total costs.
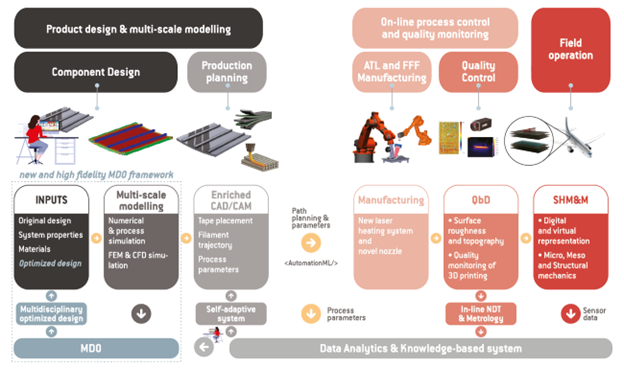
This WP is aimed at the development of numerical models of ATL and FFF processes for designing the optimum strategy for manufacturing the multifunctional composite representative prototypes expected by DOMMINIO. In the frame of this WP we created the numerical model enabling simulating both ATL and FFF processes, by combining advanced simulation tools based on the use of model order reduction and advanced machine learning techniques able to produce parametric solutions of parametrized models, from which simulation, optimization, inverse analysis, uncertainty propagation and simulation-based control perform under the stringent real-time constraints. Among the last, the employed techniques concerned TDA – topological data analysis- able to extract compact and efficient descriptors of tape surfaces, with a high impact on the interface quality. The works in progress concerning FFF will focus on the employ of CNN (convolutional Neural Networks), GNN (Graph Neural Networks) and rNN (recurrent Neural Networks) physically informed for predicting the extruded filament shape evolution.
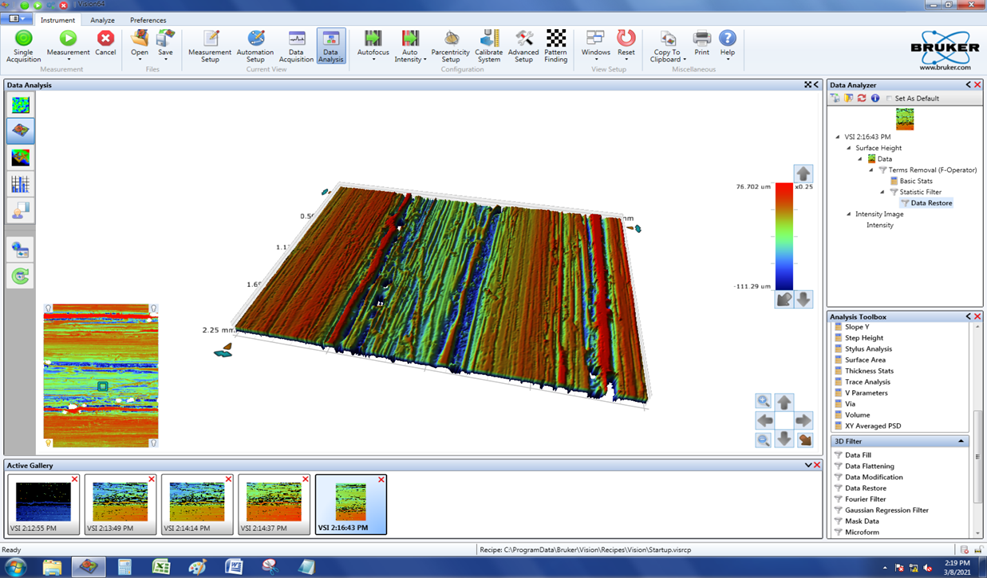
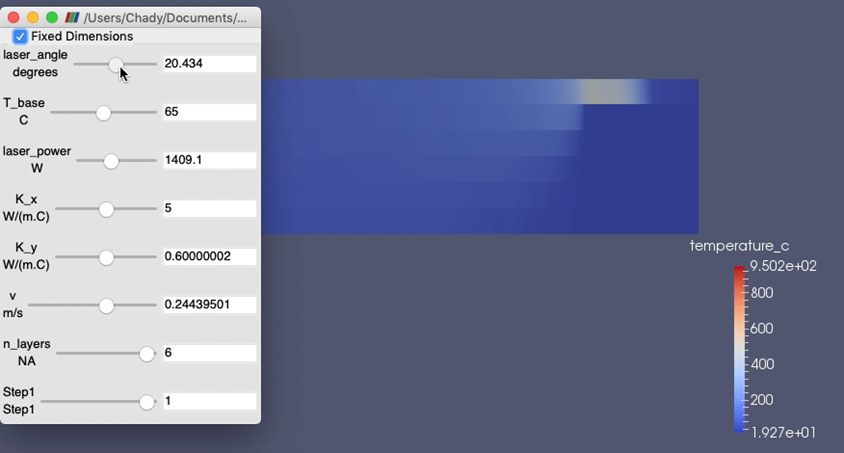
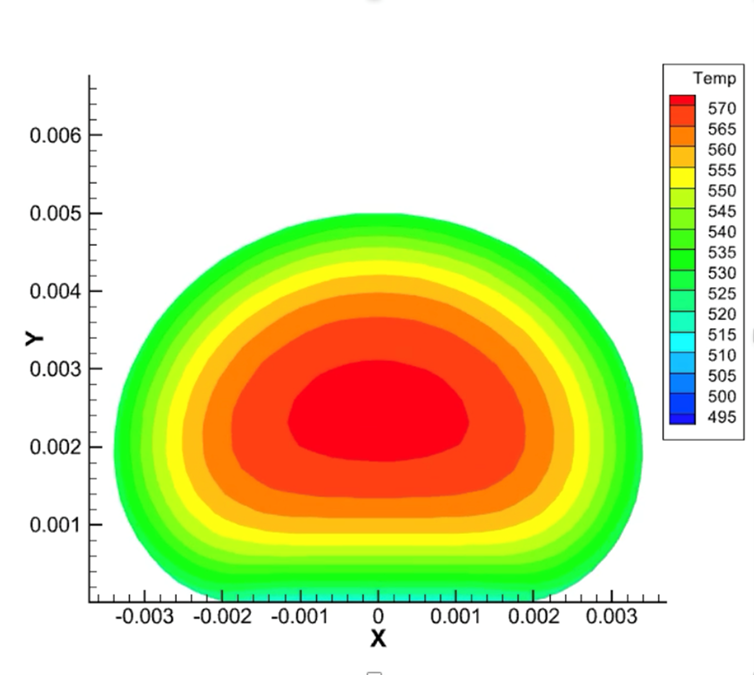
This WP is devoted to functional materials engineering and the experimental parametrization of the DOMMINIO multi-stage manufacturing process.
Materials development
The thermoplastic composite filaments for FFF have been developed and characterized. This includes the filaments reinforced with continuous carbon fiber (cCF) for structural purposes, filament reinforced with continuous carbon nanotube fiber (cCNT) for SHM, and filament filled with magnetic nanoparticles (MNP´s) for debonding on demand (Figure 1).
AFP Processing window
The AFP cell has been equipped with “heated table” for better temperature control. Fine tuning of the process parameters is currently in progress, Figure 3.
FFF Processing window
A laser heating system has been mounted in the FFF cell, Figure 4. Trials for printing the filaments reinforced with cCF are ongoing, hybrid samples combining neat and cCF reinforced filaments are currently being fabricated. Activities on this task are also being used to support the FFF modelling activities in the frame of “Process numerical modelling and multifunctional prototypes design”, as described above.
Disassembly Processing window
The induction heating apparatus for testing developed nanocomposite filaments based on the hysteresis loss mechanism heating has been set up for the initial definition of the processing window for de-bonding on demand (Figure 5). PEKK nanocomposites with 2.5-10% wt. MNPs concentration were tested.
This WP is focused on the development simulation models and digital tools to enable the detailed micro and meso-mechanical failure analysis and detecting/locating damages in structural composites. Multiscale modelling techniques are first used for developing a digital twin of the mechanical behavior of laminates. Deep learning models (Recurrent Neural Networks) are used to generate surrogates of the mechanical behavior in a multiscale analysis. Deep learning models (Convolutional Neural Networks) are also used to detect the presence of damages with information gathered with advanced CNT sensors integrated in the structural laminate.